How we make recommendations
Our Opportunity Audits uncover problems and opportunities with digital products and services using a wide range of UX methodologies that include; UX Heuristic Reviews, Moderated Usability Testing, Data Analysis, and Ideation Workshops.
By triangulating these qualitative and quantitative sources, we provide a set of actionable recommendations, allowing the experimentation roadmap to be updated with prioritised, evidence-based problem statements and hypotheses.
Recommendations come in four flavours, determined by the volume of evidence and data gathered (leading to a higher or lower rate of confidence), and the complexity of the proposed solution.
The recommendations are put into four categories, as follows:
- Fix / JDI (“Just do it”)
- A/B/n test
- UX Prototyping
- Investigation
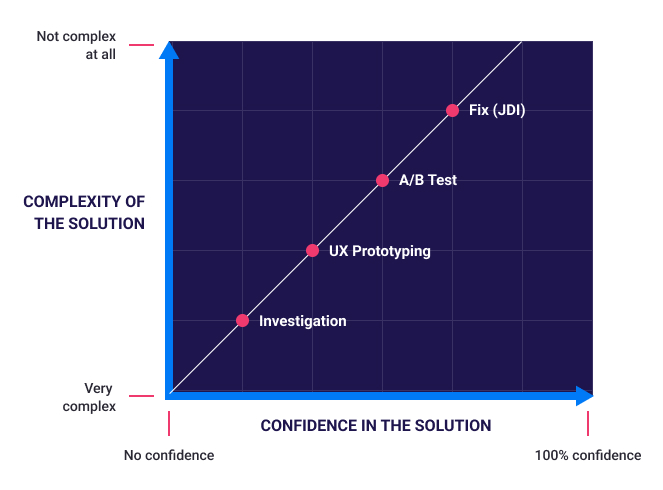
Low-fidelity prototypes and quick mockups
For smaller or simpler recommendations (which would usually fit into the JDI or A/B/n test categories), quick, low fidelity prototypes are usually sufficient.
When we have high confidence in a solution, the need for in-depth prototyping is reduced, as we can either confidently roll out the change (in the case of a JDI), or move straight to an A/B/n test with variations.
These are therefore simply used to demonstrate an idea visually. They may include very basic functionality, for instance showing the link between a couple of screens. For A/B/n tests, the proposed variations are shown.
Medium-fidelity prototypes
When making more complex or less certain recommendations, mapping out the flows into a prototype is essential.
This is useful to create a proof of concept, and may highlight unforeseen issues, reducing the need for QA, amends, and dev-time later on in the process.
Prototypes of this fidelity also provide us with a limited ability to test with users. For instance, we can quickly test variations and iterations with thousands of respondents using reaction testing, first-click testing, and preference testing. Tools such as UsabilityHub are perfect for this.
This enables some early insight and may flag potential problems, allowing our recommendations to be evidence-based and data-driven as far as possible.
High-fidelity prototypes
Occasionally it is useful to more fully prototype the entire idea or flow, in which case an accurate prototype is needed to simulate a realistic experience.
For instance, in the case of re-designing an onboarding flow that includes ID verification, it may be difficult to fully appreciate potential pain points, or usability issues, without a more functional and complete flow.
Tools such as ProtoPie and Anima allow us to create more elaborate prototypes, with form field entries, use of the device camera, and even realistic 2-factor authentication using cross-device functionality.
More sophisticated prototypes allow us the opportunity for more realistic usability testing, meaning the insights we get are more reliable and likely applicable to the “real version”.
Live prototypes
Sometimes, there are just too many hover states, potential paths, or interactions on a page for a UX prototype made with tools like Figma to be a practical solution. In these cases, live prototyping is a great option.
We create a client-side A/B test variation, by actually developing the potential solution in code, allowing us to generate a fully working prototype on top of the live website. By setting a cookie on the recruited users’ phone or laptop, we’re then able to test the prototype via a unique URL.
Moderated usability testing is then possible to the same high standard as testing on the live site, retaining more natural, realistic user behaviour, and avoiding the need for highly specific tasks and setup.
A typical use-case is testing search or navigation features, where even a high-fidelity prototype would likely struggle to account for every category, journey, and path; in a live prototype all of these can easily be tested, and testing can remain exploratory.
An added advantage of this process is that, if there are only minor changes to make based on usability testing, it is much quicker to roll out an A/B/n test as the experiment is already built and ready to launch on the experimentation platform.
Final thoughts
It is worth noting that none of these options test at scale, so A/B/n testing the final experience after gathering insights from prototypes is crucial.
As time investment and risk typically increase with fidelity, it is critical to have prior evidence before planning or building out more complex ideas to ensure changes are likely to have a significant positive impact.
A clear understanding of the problem is vital before searching for solutions, so triangulating qualitative and quantitative data from multiple sources remains as important as ever.
Prototyping is a powerful tool when used right, and choosing the right type of prototype requires thought and consideration. Different ideas and experiments benefit from different types of prototype, so a balance of value provided and time required is key.
To learn more about prototyping, our work, and how we can supercharge your testing programme, reach out to us on LinkedIn or get in touch.