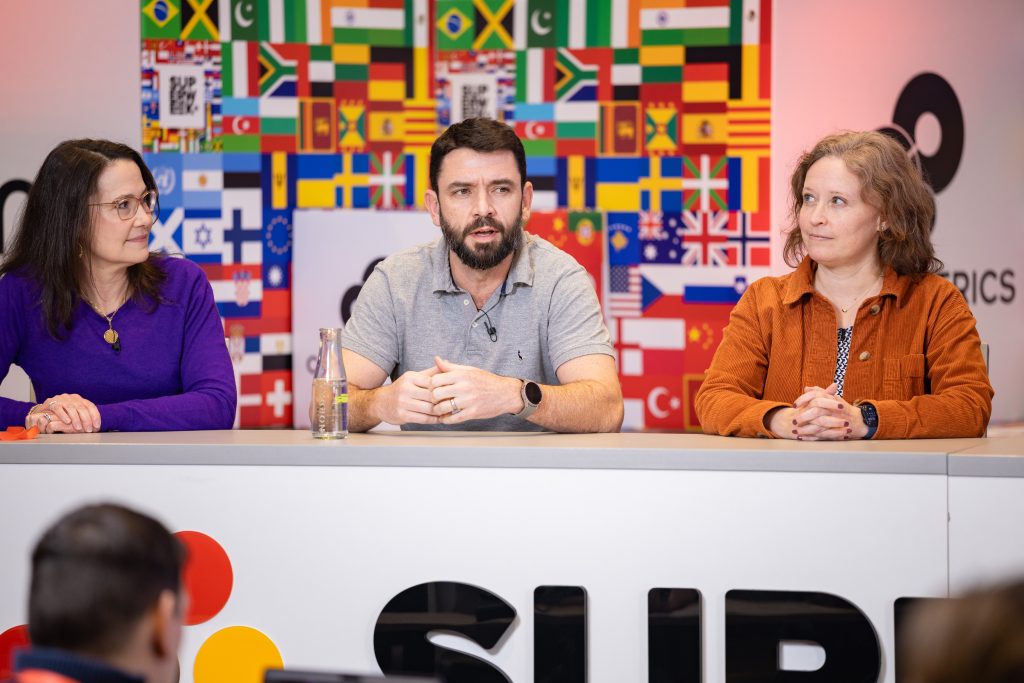
Data and analytics have always been fundamental to AB Testing/Optimisation, but as the scale of Experimentation programmes continues to grow, it is becoming more important than ever.
At this year’s Superweek in Hungary, discussions highlighted a crucial shift: data is no longer just a tool for designing and assessing experiments, it’s becoming the bridge between experimentation and real business outcomes.
Connected data and actionable insights are key to clearer impact measurement, product development risk and cost management, and driving wider business outcomes.
This shift opens up possibilities to enable low-risk innovation, personalise more effectively, and automate more intelligently.
Reflecting on My Journey with Data and Analytics
I started my digital career in web analytics nearly 20 years ago, where my work revolved around tracking performance, understanding user behaviours, and reporting key metrics.
As MarTech evolved, my role expanded beyond analytics, incorporating a broader range of tools and strategies to drive insights and impact.
This eventually led me to conversion rate optimisation (CRO), where analytics was critical, but often misused. Many relied on funnel drop-offs to identify ‘problem areas’ and then turned to intuition for solutions. While this sometimes delivered quick wins, it rarely tackled deeper, systemic issues.
Learning to Focus on the Right Problems
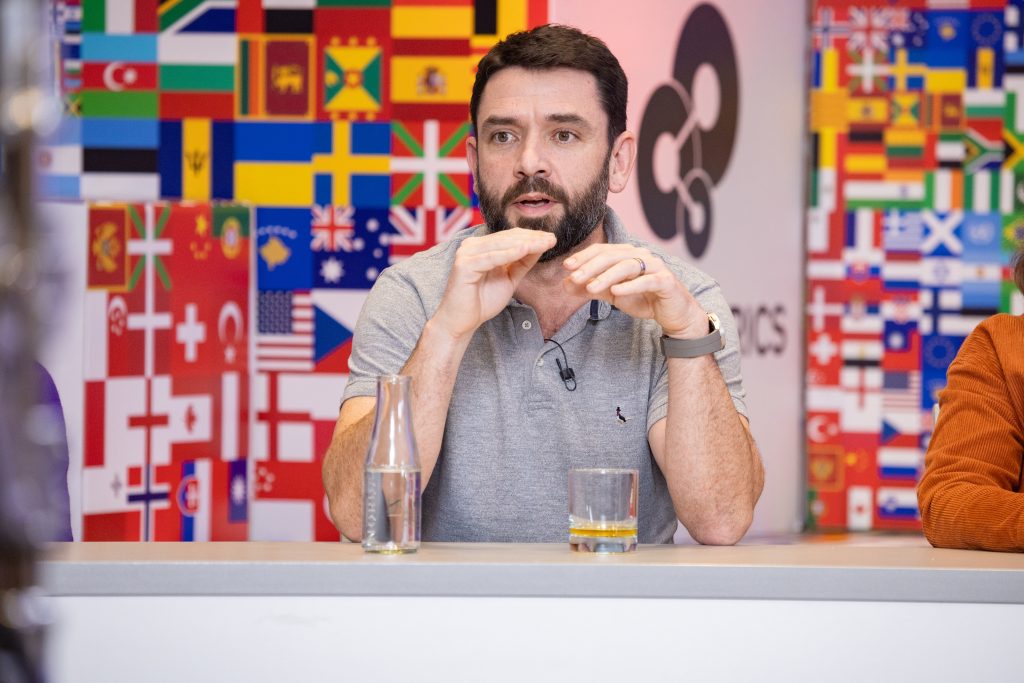
Over recent years, my colleagues at Creative CX (CCX) have helped me develop a much deeper understanding of the role data plays in problem discovery. I’ve learned that data isn’t just about measurement—it’s a crucial tool in identifying the right problems to solve before considering potential solutions.
At CCX, we don’t just analyse numbers; we connect data to qualitative research and behavioural insights to uncover the “why” behind customer challenges. The qualitative component helps us understand the reasons behind user behaviour, while the quantitative data allows us to measure how many people are affected and estimate the likely impact on both the user and the business. This approach ensures that our experimentation efforts are focused on addressing meaningful, high-impact issues rather than just surface-level symptoms.
By embedding data-driven problem discovery into our work, we ensure that our solutions are more targeted, effective, and aligned with both customer needs and business goals. This shift from diagnosing problems to truly understanding them has been transformative.
Experimentation at Scale
Experimentation at Scale has broadened our remit beyond isolated touchpoints. We’re looking at the entire customer journey (online & offline), collaborating with teams across organisations to connect insights from product, marketing, customer success, and beyond. One of the interesting developments in this evolution is our closer collaboration with data science teams. Many have already built separate models for back-end algorithm changes, feature flagging, and advanced data processing. They also provide us with rigorous propensity models that help drive data-informed decisions and personalisation strategies. This integration, alongside other advancements, is helping us unlock more impactful experimentation opportunities.
Additionally, experimentation is now taking place across various environments, from front-end interactions to complex back-end systems – and offline environments too. To maximise the value of these efforts, we are focused on connecting experimentation activities in data warehouses and CDPs, ensuring that insights are unified and actionable across the business.
By embedding these capabilities into our experimentation framework, we are making data-driven optimisation more rigorous, scalable, and impactful than ever before.
Deeper integration of back-end systems and richer data sources hold valuable insights that enhance experimentation in several ways:
- Better understand the customer journey: both the initial sales journey and the ongoing lifetime relationship.
- Connect activities to outcomes: e.g. operational costs, customer retention, and lifetime value.
- Leverage advanced models: incorporate sophisticated models, such as propensity scoring, to refine decision-making and improve personalisation.
- Improve personalisation: create more tailored customer experiences that drive engagement and loyalty.
- Scale automation: automate processes to allow organisations to optimise at scale, with precision and efficiency.
A New Era for Analytics and Experimentation
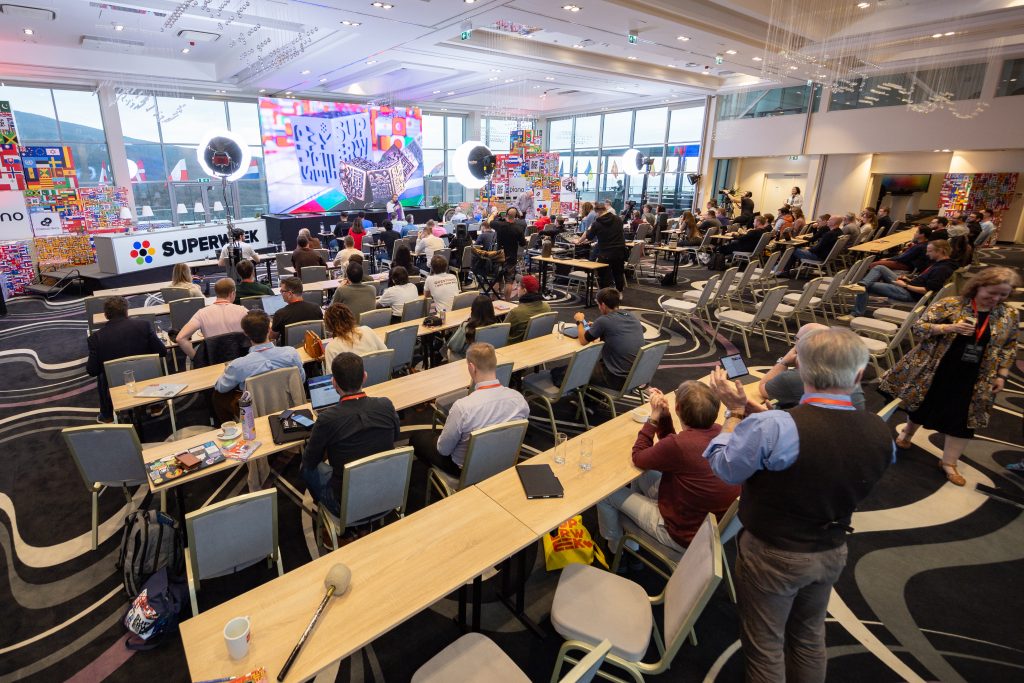
Superweek was a reminder of how far we’ve come in the world of analytics and experimentation—and how much potential still lies ahead. The role of data has evolved from simply informing decisions to driving comprehensive, connected insights that span the entire business.
As we embrace these new opportunities, we can:
- Show value more clearly by linking experimentation to key business outcomes.
- Deliver personalised experiences that delight customers and meet their unique needs.
- Automate processes to help organisations scale their efforts more effectively.
The future isn’t just about running tests; it’s about leveraging experimentation to deliver meaningful value for both customers and businesses. By connecting data, outcomes, and teams, we’re unlocking a new era of innovation. I’m excited to see where it takes us.